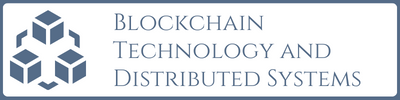
This research paper delves into the realm of predictive modelling for insurance pricing, focusing on a comparative analysis of various machine learning approaches. The study scrutinizes the accuracy, interpretability, and scalability of these methods to offer insights into their effectiveness within the insurance domain. Leveraging extensive datasets, diverse machine learning algorithms are examined, including decision trees, random forests, gradient boosting machines, neural networks, and support vector machines. Each approach is rigorously evaluated based on its predictive performance, transparency of decision-making processes, and computational efficiency. Through empirical analysis and statistical comparisons, this paper illuminates the strengths and limitations of different techniques, providing valuable guidance for insurance companies seeking optimal pricing strategies.