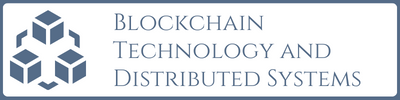
The rapid evolution of digital technologies has necessitated a paradigm shift in organizational frameworks, particularly within the banking and financial sector. This research paper examines the synergistic integration of Kanban methodologies and artificial intelligence (AI) as a transformative approach for enhancing operational efficiency during the digital transformation of Agile enterprises. The study posits that Kanban, a visual workflow management tool rooted in lean principles, can be significantly augmented by AI capabilities to optimize process automation, task management, and cross-departmental collaboration.
In an era where agility is paramount, organizations are increasingly adopting Agile methodologies to foster flexibility and responsiveness to market dynamics. However, the inherent complexities and interdependencies within financial services necessitate a more structured approach to workflow management. The traditional Kanban framework, while effective in visualizing work-in-progress and limiting work in progress (WIP), often encounters challenges in scalability and adaptability within the context of diverse financial processes. The integration of AI technologies can address these challenges by providing advanced analytics, predictive capabilities, and intelligent automation, thereby enhancing the decision-making process and streamlining operations.
This paper explores various dimensions of how Kanban, when coupled with AI, can facilitate a more efficient digital transformation journey. It delves into the optimization of process automation, where AI algorithms can analyze workflow patterns, identify bottlenecks, and suggest real-time adjustments to enhance throughput. Additionally, task management is examined through the lens of AI-driven prioritization and resource allocation, which can lead to more informed decision-making and improved productivity across teams.
Cross-departmental collaboration, a critical success factor in Agile enterprises, is also scrutinized. The interplay between Kanban boards and AI can promote transparency and alignment among various departments, fostering a culture of collaboration and shared accountability. The research emphasizes that the dual implementation of Kanban and AI can not only enhance operational efficiency but also align strategic objectives with day-to-day operations, thereby contributing to a holistic approach to digital transformation.
In addition to theoretical explorations, this study incorporates empirical evidence from case studies within the banking sector, illustrating successful applications of Kanban and AI in optimizing process workflows. The findings indicate that organizations leveraging this integrated approach have witnessed significant improvements in key performance indicators (KPIs), including cycle time reduction, enhanced customer satisfaction, and increased adaptability to changing market conditions.
However, the implementation of this integrated framework is not without challenges. The paper identifies potential obstacles, including resistance to change, the need for cultural shifts within organizations, and the necessity for appropriate technology infrastructure. The discussion culminates in practical recommendations for organizations seeking to adopt this innovative approach, emphasizing the importance of fostering a culture of continuous improvement and iterative learning.