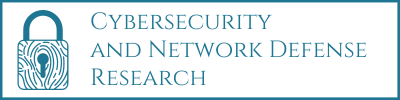
In the rapidly evolving landscape of mobile product development, product managers face increasing complexity in aligning product features with ever-changing user preferences and technological advancements. The traditional methods of feature prioritization and user experience (UX) optimization, often guided by intuition and limited data analysis, struggle to keep pace with the vast and dynamic streams of user behavior data generated by mobile applications. This paper investigates how artificial intelligence (AI), specifically machine learning (ML), can transform product management strategies to make them more data-driven, customer-centric, and responsive to real-time user needs in mobile product development. By leveraging AI-driven analytics, product managers can unlock deeper insights into user behavior patterns, preferences, and pain points, enabling more informed and agile decision-making.
The paper begins by reviewing the existing approaches to product management in mobile applications and the growing demand for enhanced customer-centricity in the design and development processes. It highlights the limitations of traditional methods, which often fail to adequately capture and process the scale of user data required for optimal decision-making. In contrast, AI-driven product management systems can ingest and analyze vast amounts of real-time data, providing actionable insights for improving product features and user satisfaction.
Next, the paper focuses on machine learning's specific role in feature prioritization, a critical aspect of mobile product development. Traditional feature prioritization methods, such as the MoSCoW (Must have, Should have, Could have, and Won’t have) method, Kano model, and cost-benefit analysis, though effective to some extent, often rely on static inputs and do not dynamically adapt to evolving user needs. This paper demonstrates how machine learning algorithms, including classification models, clustering techniques, and predictive analytics, can automate and optimize feature prioritization by analyzing historical and real-time user data. By employing techniques like natural language processing (NLP) for sentiment analysis, unsupervised learning for user segmentation, and reinforcement learning for adaptive decision-making, product managers can rank features more accurately based on user preferences, market trends, and potential business impact.
Another essential focus of this paper is user experience optimization, which is increasingly recognized as a key differentiator in the competitive mobile application market. The research explores how AI-powered UX analytics tools can continuously monitor user interactions, identify friction points, and provide predictive insights into user satisfaction. Machine learning models, such as decision trees, neural networks, and collaborative filtering, are discussed for their effectiveness in optimizing user journeys and personalizing content delivery. This paper further emphasizes the importance of using AI to detect anomalies in user behavior, anticipate potential churn, and implement corrective measures to retain users.
The paper also presents case studies and real-world applications of AI-driven product management in successful mobile products. These examples illustrate how organizations have implemented machine learning algorithms to prioritize features based on real-time user feedback and optimize UX for enhanced engagement and retention. Key performance indicators (KPIs), such as feature adoption rates, user satisfaction scores, and churn reduction, are used to measure the success of AI-driven strategies. Additionally, the role of explainable AI (XAI) in product management is addressed, ensuring transparency and trust in AI decision-making processes.
Moreover, this paper delves into the challenges of integrating AI into mobile product management workflows. These challenges include data quality and accessibility, the need for specialized AI talent, the complexity of model interpretability, and ethical considerations surrounding user data privacy. The paper provides a framework for overcoming these obstacles, proposing solutions such as data governance protocols, collaboration between data scientists and product managers, and ensuring compliance with privacy regulations like GDPR and CCPA.
Finally, the paper concludes by discussing future directions for AI-driven product management strategies, particularly in mobile product development. The increasing ubiquity of AI tools, such as deep learning and reinforcement learning, in analyzing user data will further enhance the precision and agility of product decisions. Additionally, advancements in real-time data analytics and AI explainability will continue to elevate the role of AI in driving customer-centric product strategies. The paper argues that the convergence of AI and product management represents a paradigm shift in how mobile applications are developed, delivering personalized and dynamic experiences tailored to individual users.
By leveraging machine learning for feature prioritization and user experience optimization, product managers can harness the full potential of user data to create products that are not only more aligned with user needs but also adaptive to market changes. This approach not only increases the efficiency of product management but also enhances user satisfaction and loyalty, providing a competitive edge in the fast-paced mobile application industry.