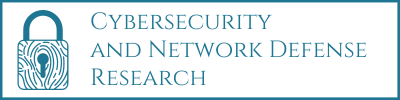
The increasing development of cyber threats has urged the establishment of efficient Intrusion Detection Systems that can predict and mitigate these attacks. With the ability to predict future threats and implement mechanisms, machine learning techniques offer excellent solutions in making IDS more intelligent, autonomous, and adaptive for future threats. This paper elaborates the application of multiple ML-based techniques to detect and predict cyber threats within IDS systems, analyzing the effectiveness and performance as well as discussing the limitation areas. Comparisons among supervised, unsupervised, and hybrid models were presented to show each's capability in enhancing accuracy with an IDS and reduction of response times.