AI-Powered Solutions for Advanced In-Car Navigation Systems
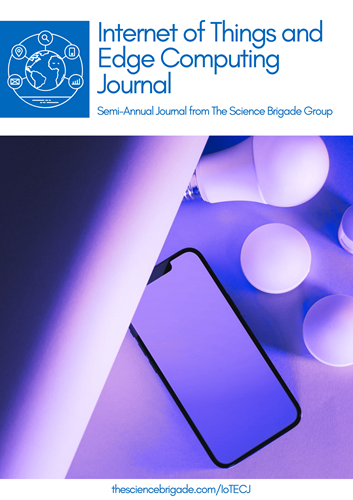
Abstract
Every driver expects the in-car navigation system to provide them with the most convenient and fastest routes, particularly in unknown terrain. Current travel times and state-of-the-art traffic information play a decisive role here. In Germany, around half of drivers receive comprehensive route guidance via route guidance software; a significant percentage consult apps on their smartphones, primarily to find their way around cities. Reprogramming the route on the move is standard for many. AI-supported route computing has been attracting increasing interest in recent years with the advance of software algorithms and navigation devices. It can better evaluate large amounts of data and thus have a greater predictive effect to provide even more precise information about estimated arrival times.
Such systems can also provide advanced route planning that not only considers the current traffic situation but also the weather, vehicle charge status, charging infrastructure, etc. In recent years, AI has made some revolutionary breakthroughs in the domain of advanced driving solutions. It has made driving safer and more efficient. Overcoming the limitations of traditional routing algorithms, current research focuses on developing systems that use artificial intelligence to support mobility concepts and individual route planning with advanced route criteria. A lot of research has been done to develop energy-efficient and eco-friendly automotive systems. Vehicles equipped with range estimation technologies powered by AI can provide information about the battery life needed to complete a given trip. The system also directs vehicles to the next available battery charging stations. The aim is to present increased accuracy of route predictions by utilizing artificial intelligence techniques and various clustering algorithms.
References
- Tamanampudi, Venkata Mohit. "Automating CI/CD Pipelines with Machine Learning Algorithms: Optimizing Build and Deployment Processes in DevOps Ecosystems." Distributed Learning and Broad Applications in Scientific Research 5 (2019): 810-849.
- Singh, Jaswinder. "Deepfakes: The Threat to Data Authenticity and Public Trust in the Age of AI-Driven Manipulation of Visual and Audio Content." Journal of AI-Assisted Scientific Discovery 2.1 (2022): 428-467.
- Machireddy, Jeshwanth Reddy. "Revolutionizing Claims Processing in the Healthcare Industry: The Expanding Role of Automation and AI." Hong Kong Journal of AI and Medicine 2.1 (2022): 10-36.
- S. Kumari, “Kanban-Driven Digital Transformation for Cloud-Based Platforms: Leveraging AI to Optimize Resource Allocation, Task Prioritization, and Workflow Automation”, J. of Artificial Int. Research and App., vol. 1, no. 1, pp. 568–586, Jan. 2021
- Tamanampudi, Venkata Mohit. "AI and DevOps: Enhancing Pipeline Automation with Deep Learning Models for Predictive Resource Scaling and Fault Tolerance." Distributed Learning and Broad Applications in Scientific Research 7 (2021): 38-77.