Machine Learning for Real-Time Traffic Management in Smart Cities
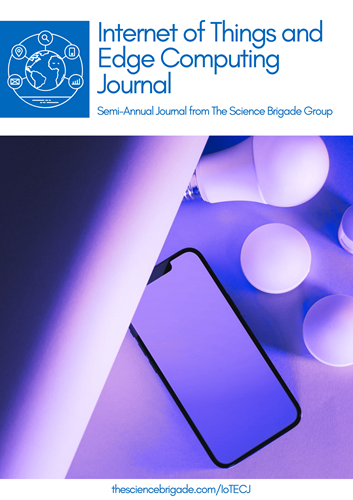
Abstract
Urbanization and population displacement to cities highlight the increasing importance of providing efficient transportation systems. Congestion has manifold effects on city dwellers, from wasting time and fuel to reducing mean speed, increasing environmental pollution, and various health hazards. Many cities across the globe emphasize initiatives that aim to adopt smart city solutions, including the alteration of the built environment, smart infrastructures, passive systems, and the provision of real-time information services to enhance citizens’ well-being. A smart city is a euphemism for a city of the digital era. Among other facilities, transportation plays a vital role in reshaping a smart city. To improve the functioning of the existing transportation system, advanced traffic management solutions have been promoted. Operations research and management theory, along with information technology, have been successfully used in traffic management systems. The development of new machine learning algorithms has provided opportunities to approach this problem from different perspectives in achieving optimal vehicle coordination at cross-sections in urban traffic settings.
References
- Tamanampudi, Venkata Mohit. "Automating CI/CD Pipelines with Machine Learning Algorithms: Optimizing Build and Deployment Processes in DevOps Ecosystems." Distributed Learning and Broad Applications in Scientific Research 5 (2019): 810-849.
- J. Singh, “Understanding Retrieval-Augmented Generation (RAG) Models in AI: A Deep Dive into the Fusion of Neural Networks and External Databases for Enhanced AI Performance”, J. of Art. Int. Research, vol. 2, no. 2, pp. 258–275, Jul. 2022
- Machireddy, Jeshwanth Reddy. "Data-Driven Insights: Analyzing the Effects of Underutilized HRAs and HSAs on Healthcare Spending and Insurance Efficiency." Journal of Bioinformatics and Artificial Intelligence 1.1 (2021): 450-470.
- S. Kumari, “Kanban and AI for Efficient Digital Transformation: Optimizing Process Automation, Task Management, and Cross-Departmental Collaboration in Agile Enterprises”, Blockchain Tech. & Distributed Sys., vol. 1, no. 1, pp. 39–56, Mar. 2021
- Tamanampudi, Venkata Mohit. "Natural Language Processing in DevOps Documentation: Streamlining Automation and Knowledge Management in Enterprise Systems." Journal of AI-Assisted Scientific Discovery 1.1 (2021): 146-185.