AI-Powered Optimization of Insurance Premium Calculation
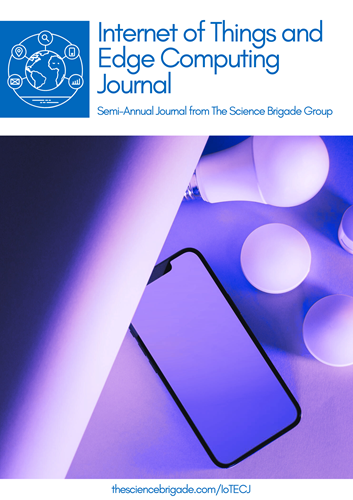
Abstract
Artificial intelligence (AI) has already made a prominent entrance into various fields such as natural language processing, robotics, medicine, transportation, and finance. In the area of finance, AI increasingly appears as a mandatory skill to learn since very often AI solutions are superior to traditional methods. A couple of benefits can be named: AI can simulate human-like thinking about certain issues, and AI excels humans in handling complex data sets. One industry in which AI solutions are 'bleeding-edge' is insurance. In insurance markets, huge amounts of data are collected to calculate the premium for a contract. However, the premiums are under criticism since there is a lack of transparency throughout the pricing process and the pricing itself.
One can talk about two sub-questions guiding our research. First, is it possible to apply AI methods to calculate more reliable and fairer premium rates? The second sub-question advises us on how to model the premium 'AI-wise' the best. In our research study, we will strive to answer these two sub-questions and thereby the main research question. This paper is organized as follows. In Section 2, we focus on the theory of individual components of our research, and after that, in Section 3, we connect these theories to provide a solution to the described problem of over-simplification of premiums. In Section 4, we provide a demonstration of our approach on some specific data, and finally, in Section 5, we discuss our findings.
References
- S. Kumari, “Cybersecurity in Digital Transformation: Using AI to Automate Threat Detection and Response in Multi-Cloud Infrastructures ”, J. Computational Intel. & Robotics, vol. 2, no. 2, pp. 9–27, Aug. 2022
- Tamanampudi, Venkata Mohit. "Automating CI/CD Pipelines with Machine Learning Algorithms: Optimizing Build and Deployment Processes in DevOps Ecosystems." Distributed Learning and Broad Applications in Scientific Research 5 (2019): 810-849.
- Machireddy, Jeshwanth Reddy. "Data-Driven Insights: Analyzing the Effects of Underutilized HRAs and HSAs on Healthcare Spending and Insurance Efficiency." Journal of Bioinformatics and Artificial Intelligence 1.1 (2021): 450-470.
- Singh, Jaswinder. "Social Data Engineering: Leveraging User-Generated Content for Advanced Decision-Making and Predictive Analytics in Business and Public Policy." Distributed Learning and Broad Applications in Scientific Research 6 (2020): 392-418.
- Tamanampudi, Venkata Mohit. "AI and DevOps: Enhancing Pipeline Automation with Deep Learning Models for Predictive Resource Scaling and Fault Tolerance." Distributed Learning and Broad Applications in Scientific Research 7 (2021): 38-77.
- J. Singh, “Combining Machine Learning and RAG Models for Enhanced Data Retrieval: Applications in Search Engines, Enterprise Data Systems, and Recommendations ”, J. Computational Intel. & Robotics, vol. 3, no. 1, pp. 163–204, Mar. 2023.
- Tamanampudi, Venkata Mohit. "AI Agents in DevOps: Implementing Autonomous Agents for Self-Healing Systems and Automated Deployment in Cloud Environments." Australian Journal of Machine Learning Research & Applications 3.1 (2023): 507-556.