AI-Based Real-Time Emergency Response Systems for Autonomous Vehicles
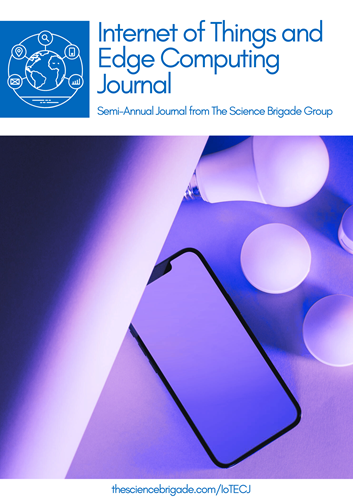
Abstract
The deployment of autonomous vehicles (AVs) has increased considerably in recent years. Their increased adoption in various domains and industries underscores the need to ensure their performance and reliability in the long term. Vehicular accidents necessitate the development of emergency response systems (ERS) that are effective, real-time, and reliable. A vigorous ERS promises improved safety, better policymaking, and public safety improvements. As critical AI methods, intelligent systems have shown promise in marshalling the complex structure of vehicular control operations and processing multimodal communication flows. These AI algorithms result in a unique approach to absorb information from various scenarios and develop operations and decision protocols in real-time.
Although an emergency for an AV can arise from a vehicular accident, terrorist attack, or natural disaster events, the research proposition here explores the advantages of having an AI-based ERS in an AV to prevent mishaps. The ERS can help return safety levels to normal by evacuating passengers from the AV, coordinating with local emergency aid services to reach the site immediately, delivering real-time AV status information to emergency operations, and accessing optimal avenues for an AV to go in the presence of vegetation blockage and wildfire. The concepts of an AV and the ERS are presented. In the next part, perceptions of a robust AV and its conscientious role for various stakeholders are recognized as international law. This paper is organized as follows. Subsequently, we describe earlier related work, the context of this paper, the creation of the AV, and the aims of the ERS. Finally, the first and second aspects of an EV-ERS are summarized in this essay.
References
- Tamanampudi, Venkata Mohit. "Automating CI/CD Pipelines with Machine Learning Algorithms: Optimizing Build and Deployment Processes in DevOps Ecosystems." Distributed Learning and Broad Applications in Scientific Research 5 (2019): 810-849.
- Pal, Dheeraj Kumar Dukhiram, et al. "AIOps: Integrating AI and Machine Learning into IT Operations." Australian Journal of Machine Learning Research & Applications 4.1 (2024): 288-311.
- Pasupuleti, Vikram, et al. "Enhancing supply chain agility and sustainability through machine learning: Optimization techniques for logistics and inventory management." Logistics 8.3 (2024): 73.
- J. Singh, “Robust AI Algorithms for Autonomous Vehicle Perception: Fusing Sensor Data from Vision, LiDAR, and Radar for Enhanced Safety”, Journal of AI-Assisted Scientific Discovery, vol. 4, no. 1, pp. 118–157, Apr. 2024
- Alluri, Venkat Rama Raju, et al. "DevOps Project Management: Aligning Development and Operations Teams." Journal of Science & Technology 1.1 (2020): 464-487.
- Machireddy, Jeshwanth Reddy. "Assessing the Impact of Medicare Broker Commissions on Enrollment Trends and Consumer Costs: A Data-Driven Analysis." Journal of AI in Healthcare and Medicine 2.1 (2022): 501-518.
- Ahmad, Tanzeem, et al. "Hybrid Project Management: Combining Agile and Traditional Approaches." Distributed Learning and Broad Applications in Scientific Research 4 (2018): 122-145.
- Tamanampudi, Venkata Mohit. "AI-Powered NLP Agents in DevOps: Automating Log Analysis, Event Correlation, and Incident Response in Large-Scale Enterprise Systems." Journal of Artificial Intelligence Research and Applications 4.1 (2024): 646-689.
- J. Singh, “The Ethical Implications of AI and RAG Models in Content Generation: Bias, Misinformation, and Privacy Concerns”, J. Sci. Tech., vol. 4, no. 1, pp. 156–170, Feb. 2023
- S. Kumari, “Optimizing Mobile Platform Security with AI-Powered Real-Time Threat Intelligence: A Study on Leveraging Machine Learning for Enhancing Mobile Cybersecurity”, J. of Art. Int. Research, vol. 4, no. 1, pp. 332–355, Jan. 2024.
- Praveen, S. Phani, et al. "Revolutionizing Healthcare: A Comprehensive Framework for Personalized IoT and Cloud Computing-Driven Healthcare Services with Smart Biometric Identity Management." Journal of Intelligent Systems & Internet of Things 13.1 (2024).
- Bonam, Venkata Sri Manoj, et al. "Secure Multi-Party Computation for Privacy-Preserving Data Analytics in Cybersecurity." Cybersecurity and Network Defense Research 1.1 (2021): 20-38.
- Tamanampudi, Venkata Mohit. "Leveraging Machine Learning for Dynamic Resource Allocation in DevOps: A Scalable Approach to Managing Microservices Architectures." Journal of Science & Technology 1.1 (2020): 709-748.