Leveraging AI for Enhanced Customer Insights in Banking
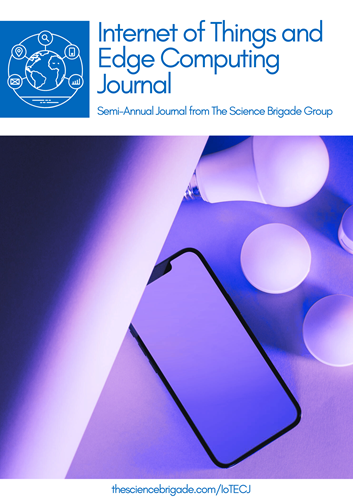
Abstract
Artificial intelligence (AI) is penetrating its way into the financial landscape, changing how banks operate, manage, and provide customer service. The purpose of AI in banking is to transform a vastly data-rich and laborious industry into a customer-centered, insights-focused field ripe for innovation. More than 92% of large banks in South Africa are banking on AI to scale their business viability and growth. A key application of AI is the ability to make intelligent predictions. While high-end analytics has been a reality in the industry, AI technologies, which include popular sub-branches such as machine learning, NLP, and so much more, are now being used to analyze patterns and develop trends more readily.
Banks have a number of AI nodes at their disposal. Among them, these five are popularly used: Natural Language Processing (NLP), Machine Learning (ML), Deep Learning, Supervised Learning, and Unsupervised Learning. Banks are becoming cognizant of AI's proficiency in customer support and interaction to the point where, within the next three years, almost 86% will be executing more marketing and sales efforts with AI. The succinct reasoning is that the quicker and more effectively banks can canvass information through vast databases for insightful, meaningful, and actionable experiences, the better they will churn out high-margin products and meet customer needs. Let's face it: taking into account the competition across operations and customer services, banks are becoming more dependent than ever on trending towards broad-spectrum AI as a key asset to bolster their capabilities and maintain the lead on business insights and decision-making. In fact, more than 90% of successful adopters of AI in their operations will integrate microservices and AI applications by 2025 in larger scale systems designed to leverage data for high-value outcomes. Investing in newer technology is what sets the ball in motion for progress and improved productivity. Are you ready to design an infrastructure for the future today? As a trustworthy banking partner with refined experience in AI-led delivery capabilities, we want you to be.
References
- Tamanampudi, Venkata Mohit. "Automating CI/CD Pipelines with Machine Learning Algorithms: Optimizing Build and Deployment Processes in DevOps Ecosystems." Distributed Learning and Broad Applications in Scientific Research 5 (2019): 810-849.
- Pasupuleti, Vikram, et al. "Enhancing supply chain agility and sustainability through machine learning: Optimization techniques for logistics and inventory management." Logistics 8.3 (2024): 73.
- Thota, Shashi, et al. "Federated Learning: Privacy-Preserving Collaborative Machine Learning." Distributed Learning and Broad Applications in Scientific Research 5 (2019): 168-190.
- J. Singh, “Advancements in AI-Driven Autonomous Robotics: Leveraging Deep Learning for Real-Time Decision Making and Object Recognition”, J. of Artificial Int. Research and App., vol. 3, no. 1, pp. 657–697, Apr. 2023
- Alluri, Venkat Rama Raju, et al. "Serverless Computing for DevOps: Practical Use Cases and Performance Analysis." Distributed Learning and Broad Applications in Scientific Research 4 (2018): 158-180.
- Machireddy, Jeshwanth Reddy. "Assessing the Impact of Medicare Broker Commissions on Enrollment Trends and Consumer Costs: A Data-Driven Analysis." Journal of AI in Healthcare and Medicine 2.1 (2022): 501-518.
- S. Chitta, S. Thota, S. Manoj Yellepeddi, A. Kumar Reddy, and A. K. P. Venkata, “Multimodal Deep Learning: Integrating Vision and Language for Real-World Applications”, Asian J. Multi. Res. Rev., vol. 1, no. 2, pp. 262–282, Nov. 2020
- Ahmad, Tanzeem, et al. "Hybrid Project Management: Combining Agile and Traditional Approaches." Distributed Learning and Broad Applications in Scientific Research 4 (2018): 122-145.
- Tamanampudi, Venkata Mohit. "CoWPE: Adaptive Context Window Adjustment in LLMs for Complex Input Queries." Journal of Artificial Intelligence General science (JAIGS) ISSN: 3006-4023 5.1 (2024): 438-450.
- Thota, Shashi, et al. "Few-Shot Learning in Computer Vision: Practical Applications and Techniques." Human-Computer Interaction Perspectives 3.1 (2023): 29-59.
- Tamanampudi, Venkata Mohit. "Leveraging Machine Learning for Dynamic Resource Allocation in DevOps: A Scalable Approach to Managing Microservices Architectures." Journal of Science & Technology 1.1 (2020): 709-748.
- J. Singh, “Autonomous Vehicle Swarm Robotics: Real-Time Coordination Using AI for Urban Traffic and Fleet Management”, Journal of AI-Assisted Scientific Discovery, vol. 3, no. 2, pp. 1–44, Aug. 2023
- S. Kumari, “Cloud Transformation for Mobile Products: Leveraging AI to Automate Infrastructure Management, Scalability, and Cost Efficiency”, J. Computational Intel. & Robotics, vol. 4, no. 1, pp. 130–151, Jan. 2024.