The Impact of AI on Organizational Change in Digital Transformation
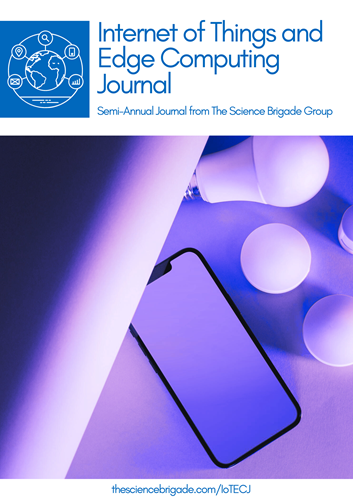
Abstract
Artificial intelligence (AI) has become a fundamental driver in the ongoing wave of digital transformation, prompting profound organizational change across multiple sectors. This research paper examines how AI influences organizational structures, workflows, decision-making processes, and cultural dynamics within organizations pursuing digital transformation initiatives. The study begins by delineating the role of AI in enabling efficient and data-driven operations, demonstrating its capacity to enhance productivity, optimize processes, and increase flexibility within corporate structures. AI systems facilitate real-time data analysis, predictive modeling, and autonomous decision-making, which collectively redefine traditional organizational practices and necessitate structural and strategic adaptations. Organizations integrating AI technologies into their digital transformation strategies frequently undergo changes that extend beyond the implementation of new technologies; they experience shifts in organizational culture, workforce composition, and skill requirements, further influenced by the need to align with ethical standards, data governance policies, and regulatory frameworks.
A significant aspect of this research is its focus on how AI reconfigures decision-making paradigms. Traditional, hierarchical decision-making models are increasingly being replaced or complemented by more decentralized, data-driven approaches that leverage AI’s analytical capabilities. Through machine learning and data analytics, AI provides insights that empower decision-makers at all organizational levels, enabling faster and more accurate responses to dynamic market conditions and customer demands. This shift not only accelerates decision cycles but also redistributes authority across different levels of an organization, contributing to a more agile and adaptable organizational model. Moreover, the study explores how AI-driven transformation affects workforce dynamics, specifically the evolving roles and responsibilities of employees. While AI-driven automation has raised concerns about job displacement, this paper also considers the emerging opportunities for reskilling and upskilling that AI integration necessitates, suggesting that a collaborative human-AI workforce can enhance organizational productivity and innovation.
The paper also examines the implications of AI on organizational culture, arguing that AI adoption in digital transformation requires a cultural shift toward data-centricity, continuous learning, and adaptability. As AI systems are integrated into core processes, organizations must foster a culture that values and utilizes data, promotes open-mindedness toward technological advancements, and encourages a proactive stance on change. This cultural shift is imperative for AI to be successfully embedded within organizations, as resistance to change can obstruct AI implementation and its intended benefits. Furthermore, the study addresses the ethical and regulatory dimensions of AI adoption, emphasizing the importance of compliance with data privacy laws, ethical standards, and the establishment of frameworks for transparent AI governance. By adopting robust AI governance mechanisms, organizations can mitigate risks associated with AI, such as biased algorithms, privacy concerns, and accountability in decision-making, thus fostering trust and credibility among stakeholders.
Finally, this research provides case studies illustrating successful organizational transformations achieved through AI integration, analyzing factors contributing to these outcomes and identifying key challenges that other organizations may face. It offers a comparative analysis across various industries to understand the diverse ways in which AI can drive digital transformation and foster organizational change. This cross-industry perspective allows for the identification of industry-specific considerations and provides actionable insights into best practices for leveraging AI in digital transformation. The paper concludes by discussing the future trajectories of AI-driven organizational change, projecting how advancements in AI technology may continue to reshape organizational structures and competitive landscapes. The findings underscore the necessity for organizations to view AI not merely as a tool but as an integral component of a larger strategic framework for digital transformation, one that is aligned with organizational goals, cultural values, and ethical principles. Ultimately, this paper contributes to the growing discourse on the impact of AI in digital transformation, providing a theoretical foundation and practical guidance for organizations navigating the complexities of AI-driven change.
Keywords
artificial intelligence, organizational change
References
- J. McCarthy, "The AI revolution: A new era of technological advancement," Communications of the ACM, vol. 62, no. 4, pp. 34-36, Apr. 2019.
- J. Manyika et al., "AI, automation, and the future of work: The impact on jobs, skills, and wages," McKinsey Global Institute, Tech. Rep. 2017.
- H. J. Kim and H. K. Kim, "Digital transformation through AI: Organizational change and implications," Journal of Business Research, vol. 120, pp. 103-115, Jan. 2020.
- A. M. DeNero, "Machine learning in organizations: Strategic implications," Harvard Business Review, vol. 97, no. 2, pp. 88-96, Mar. 2019.
- B. W. Boehm and L. Chen, "Agile software development: The transformation of software engineering," IEEE Software, vol. 35, no. 6, pp. 50-56, Nov.-Dec. 2018.
- Tamanampudi, Venkata Mohit. "Automating CI/CD Pipelines with Machine Learning Algorithms: Optimizing Build and Deployment Processes in DevOps Ecosystems." Distributed Learning and Broad Applications in Scientific Research 5 (2019): 810-849.
- S. Kumari, “AI-Powered Cloud Security for Agile Transformation: Leveraging Machine Learning for Threat Detection and Automated Incident Response ”, Distrib Learn Broad Appl Sci Res, vol. 6, pp. 467–488, Oct. 2020
- Zhu, Yue, and Johnathan Crowell. "Systematic Review of Advancing Machine Learning Through Cross-Domain Analysis of Unlabeled Data." Journal of Science & Technology 4.1 (2023): 136-155.
- Tamanampudi, Venkata Mohit. "Leveraging Machine Learning for Dynamic Resource Allocation in DevOps: A Scalable Approach to Managing Microservices Architectures." Journal of Science & Technology 1.1 (2020): 709-748.
- S. Kumari, “Kanban and Agile for AI-Powered Product Management in Cloud-Native Platforms: Improving Workflow Efficiency Through Machine Learning-Driven Decision Support Systems”, Distrib Learn Broad Appl Sci Res, vol. 5, pp. 867–885, Aug. 2019
- A. G. I. Mohammed, S. Al-Sharhan, and S. K. Wong, "Human-AI collaboration: Redefining the role of humans in AI-driven organizations," Computers in Human Behavior, vol. 117, pp. 106-120, Feb. 2021.
- R. B. R. Duflo and S. D. Smith, "Data-driven culture: How organizations can foster a data-centric mindset," Business Horizons, vol. 63, no. 4, pp. 513-522, July-Aug. 2020.
- M. C. K. Zhang, "Ethical considerations in AI: Bias, accountability, and transparency," AI & Society, vol. 35, no. 3, pp. 555-564, Sept. 2020.
- R. Kaplan and D. Norton, "The balanced scorecard: Translating strategy into action," Harvard Business Review Press, 1996.
- S. R. Quarles, "AI governance: Best practices for ethical and compliant AI systems," AI & Ethics, vol. 2, no. 2, pp. 177-186, June 2021.
- J. R. Dyer, "Digital transformation strategies: Balancing innovation and tradition," Strategic Management Journal, vol. 40, no. 9, pp. 1397-1423, Sept. 2019.
- A. B. D. K. Hsu, "AI and workforce dynamics: Opportunities for reskilling and upskilling," Journal of Organizational Behavior, vol. 42, no. 8, pp. 1098-1114, Dec. 2021.
- L. C. A. Ferreira, "Challenges of AI integration: Barriers and strategies for overcoming resistance," Journal of Technology Management, vol. 14, no. 1, pp. 45-60, Mar. 2020.
- T. P. Wu, "Future directions in AI and organizational change: Emerging trends and predictions," International Journal of Information Management, vol. 57, pp. 102-117, Oct. 2021.
- D. M. Schoemaker and R. E. B. Weller, "Decision-making in AI-enhanced organizations: A new paradigm," European Management Journal, vol. 38, no. 5, pp. 786-797, Oct. 2020.
- S. T. Yu and C. H. Liang, "The impact of AI on organizational culture and employee engagement," Journal of Business Research, vol. 116, pp. 314-322, July 2020.
- A. R. Gupta, "AI-driven digital transformation in healthcare: Benefits and challenges," Health Information Science and Systems, vol. 8, no. 1, pp. 1-10, Dec. 2020.
- V. M. G. Reyes, "Machine learning as a catalyst for digital transformation," IEEE Transactions on Engineering Management, vol. 68, no. 3, pp. 617-626, Aug. 2021.
- J. L. Davis and R. L. Wright, "Organizational change management in the era of AI," Journal of Organizational Change Management, vol. 33, no. 6, pp. 913-927, Nov. 2020.
- P. J. R. De Silva and J. J. Jones, "Artificial intelligence in strategic decision-making: A systematic literature review," Strategic Management Review, vol. 3, no. 1, pp. 35-52, Jan. 2022.